Re-Optimization: New Tasks
NextBillion.ai’s Route Optimization Flexible API offers a powerful re-optimization feature which allows the users to re-optimize a set of existing or new tasks by readjusting some variables, if needed.
Let’s suppose a business which has an unpredictable frequency of incoming orders (grocery delivery, courier services etc.) creates a route plan to execute the orders at a given point of time. By the time the plan is created, delivery vehicles are loaded with inventory, and drivers are contracted, another bunch of high priority orders is received. Now either the users can rebuild the entire optimization problem again and scrap the plan that was already in motion or just re-optimize the existing solution to accommodate the new priority orders with minimal or no changes to the original plan.
To re-optimize a routing plan the users need the previous solution created using the given constraints at the time. For successfully adding new orders/tasks to the re-optimized solution, users need to decide if the existing constraints of number of vehicles, shift timings or capacities of the vehicles, or the existing task time windows or any other variables need to be updated or not. The users are expected to rebuild the request using some of the above strategies while specifying all the new and existing tasks. Upon receiving a re-optimization request, the solver will try to accommodate the new tasks into the original solution without many changes. This way businesses can make quick or last-minute adjustments to their route planning without needing to make extravagant changes to the existing plans.
We cover this example through 3 steps - in the first step, we execute a basic route optimization request and get an original solution. In the next step, we review the new tasks received and re-model our original optimization request. In the last step, we use the solution
attribute, and re-optimize our existing solution so that all tasks are assigned. Let’s start with building the original request.
Get Started
Readers would need a valid NextBillion API key to try this example out. If you don’t have one, please contact us to get your API key now!
Setup
Once you have a valid API Key, you can start setting up the components to be used in this example. Let’s take a look at them below. Jobs & Shipments We start by defining 6 jobs and 2 shipments. For these tasks we add:
-
A unique identifier for each task
-
Location indexes for each task
-
Specify the schedule of tasks. This is done by adding time windows within which a task must be completed. We have added a 15 min time window for all tasks.
-
The actual time taken to complete the tasks once the driver/vehicle is at the task’s location i.e. the service time for each task.
-
Skills needed to perform each task
-
Pickup and Delivery amounts for all jobs
Let’s take a look at the jobs
JSON after the above properties are configured:
Vehicles
Next, we add 4 vehicles that are going to fulfill the tasks within the defined constraints. To describe the vehicles and their properties we add:
-
A unique ID for each vehicle
-
Vehicle shift time or the time window. We have added the same for both vehicles in the first step.
-
Capacity to denote the amount of load that the vehicle can take
-
Start_index to denote the point from where the vehicle would start
-
Skills for all vehicles
Once the vehicle and their properties are defined, the resulting vehicles
JSON is:
Locations
And now, lastly we would define the locations
object and add all the locations used in the problem along with a valid id
. The locations object with all the points used in this example:
Step 1: Get the original Solution
Now that we have built the basic components of an optimization problem, we can submit this request using the Optimization POST method and retrieve the solution.
Optimized POST request
Optimized POST response
Once the request is made, we get a unique ID in the API response:
Optimized GET request
We take the ID and use the Optimization GET request to retrieve the original result. Here is the GET request:
Following is the response
Following is a visual representation of the initial locations of tasks, vehicles and the routes suggested after optimization as per the given constraints.
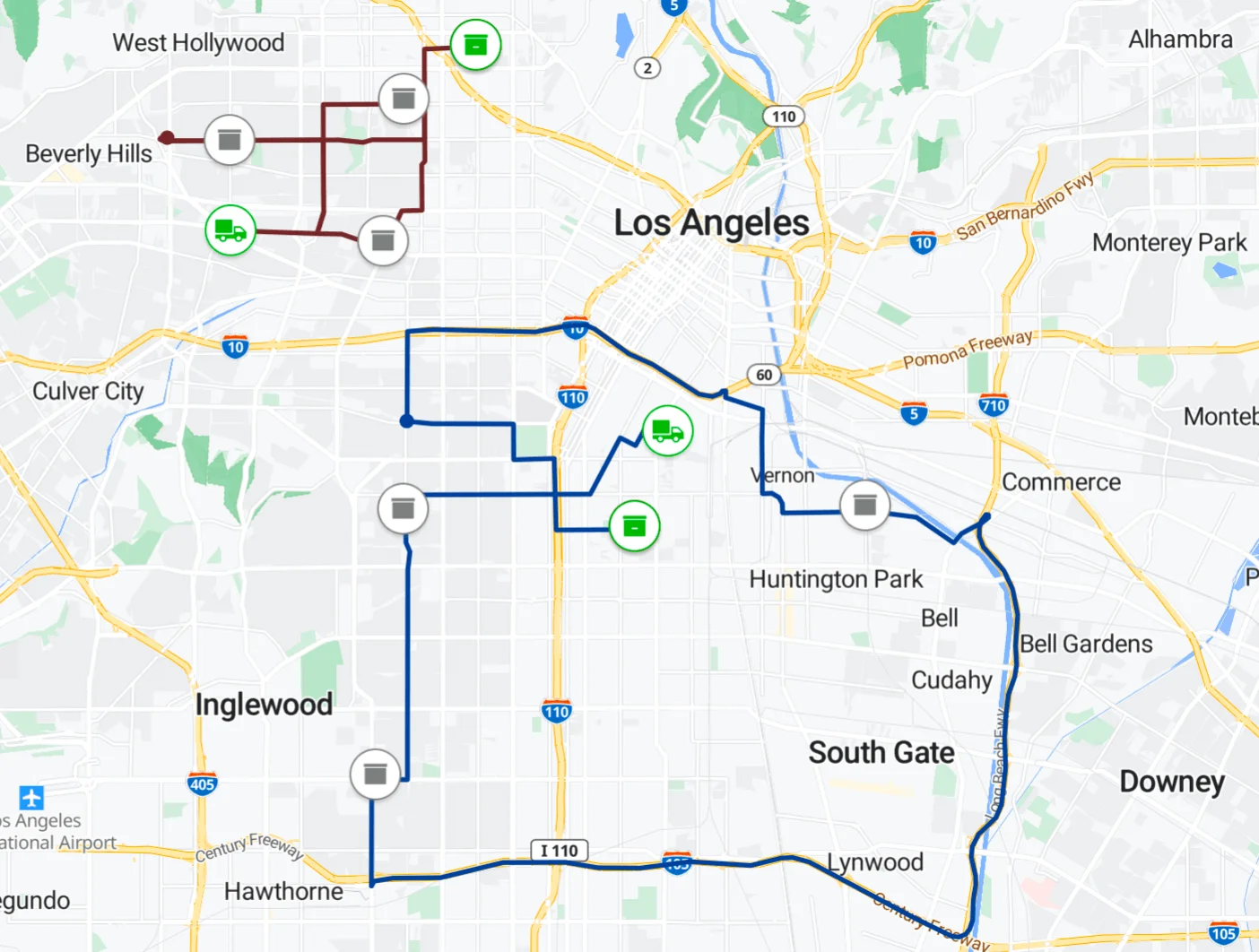
Step 2: Incorporate new changes
In the original response, we can see that there were 2 routes that took care of all the tasks and none of them remained unassigned. Readers are encouraged to study both routes and task distribution in this solution.
We will now add 4 new jobs to the mix and decide what changes to the original request are needed to ensure that all new jobs can be assigned. Let’s look at the updated components used in our original request.
New Jobs & Shipments
For the new jobs, We added jobs 4 & 5 with time windows between the existing task windows We added new jobs 6 & 7 with time windows starting after all the existing jobs got over in the original solution, but within the vehicle shift timings. These jobs should be appended to the existing routes.
Let’s look at how the job JSON looks like now with new changes:
New Vehicles
Looking at the new tasks added, there is no need to add a new skill or extend vehicle time shifts. However, we do need to update the vehicle capacity so that the new tasks can be carried out. Following is the vehicle JSON after incorporating the new changes:
New Locations
The locations
object also needs to be updated with the locations of the newly added tasks. Following is the new locations
JSON:
Step 3: Prepare for re-optimization
As a business operator when we get last minute new orders or changes to existing task time windows or any other change of sort in general, it might be in our interest to accommodate the new changes in the existing plan with minimal changes. In order to do that, we have updated our input request to add new tasks received and made other changes needed to our original optimization request to model the situation.
Now, in order to ensure that this new problem is not treated as a new request but as a re-optimization request instead, we add the original route plan to the solution
section of the input request. This will ensure minimal changes to the existing route plan while taking care of the new tasks.
Solution
We build this object by adding the route details from the original solution as it is. Following is the JSON for solution
part:
Optimization POST Request
Now let’s put all these components together and create the final POST request that we will submit to the optimizer. Please ensure to add the new jobs
, shipments
, vehicles
& locations
attributes, reflecting the updated changes, instead of the original ones. Following is the final re-optimization request.
Optimization POST Response
Once the request is made, we get a unique ID in the API response:
Optimization GET Request
We take the ID and use the Optimization GET request to retrieve the result. Here is the GET request:
Optimization GET Response
Following is the optimized route plan:
Following is a visual representation of all the task locations (new + original), location of vehicles and the routes suggested after re-optimization as per the given constraints.
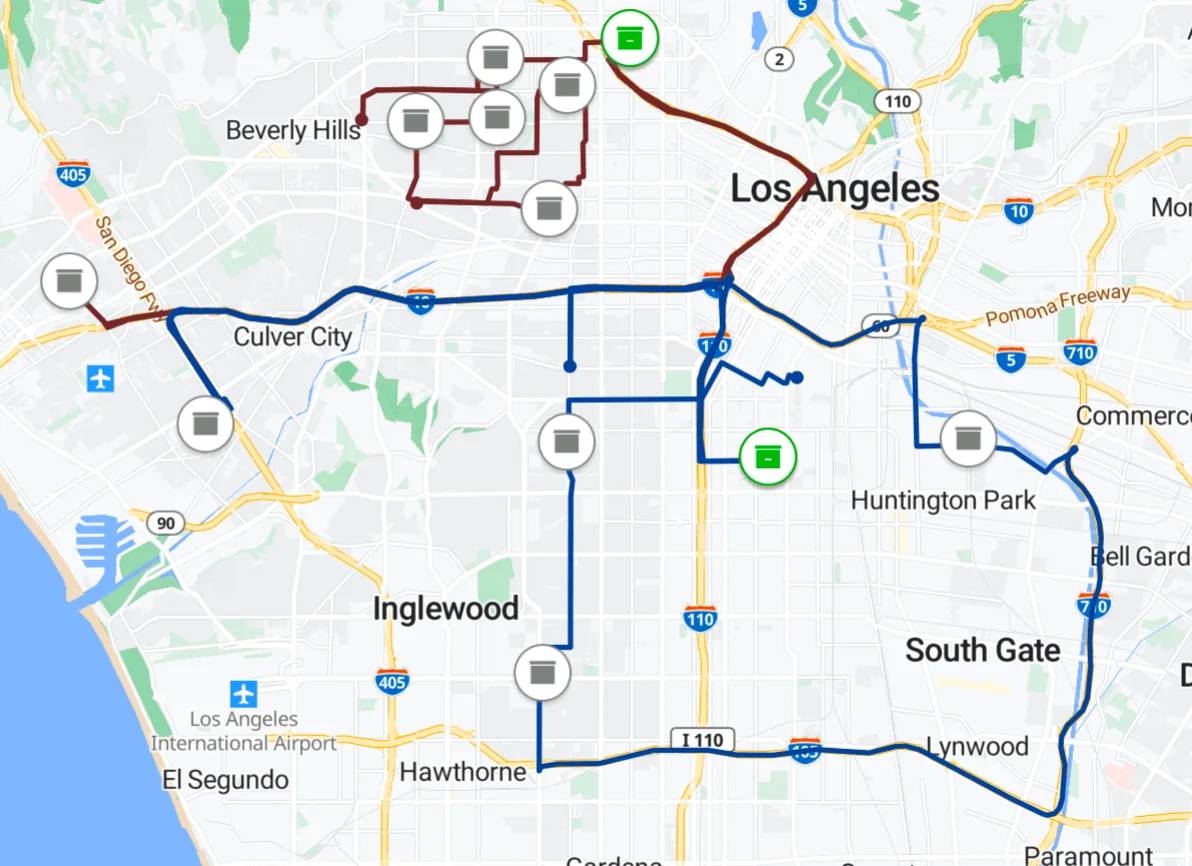
Analyzing the Solution
Looking at the new result we can observe some interesting insights:
-
All the newly added jobs - 4,5,6, & 7 were assigned within the 2 routes that were used in the original solution.
-
The new tasks (jobs 4 & 5 ) for vehicle 1 were added to the existing route without much change, while job 6 was fulfilled after all the original tasks were done owing to the time window of the job.
-
For vehicle 2, the job 7 was assigned after all the original tasks were completed owing to its time window, however important is to note that there was not much difference in the original and re-optimized until the delivery step of shipment 2 which is the last task before the vehicle heads towards the newly added task.
-
The optimizer proficiently adds all 4 tasks to the new solution with minimal changes to the original solution's existing parameters.
Above example showcases the powerful re-optimization capabilities of NextBillion.ai’s Route Optimization Flexible API. Users can leverage this feature to make last minute adjustments to their optimization plans with significant flexibility while improving overall task fulfillment. To explore this feature more, users can play around with strategies like adding more vehicles, changing the task time window and see how the API takes those changes into account without major changes to the original solution.
We hope this example was helpful. Check out some more use cases that Route Optimization Flexible API can handle for you!